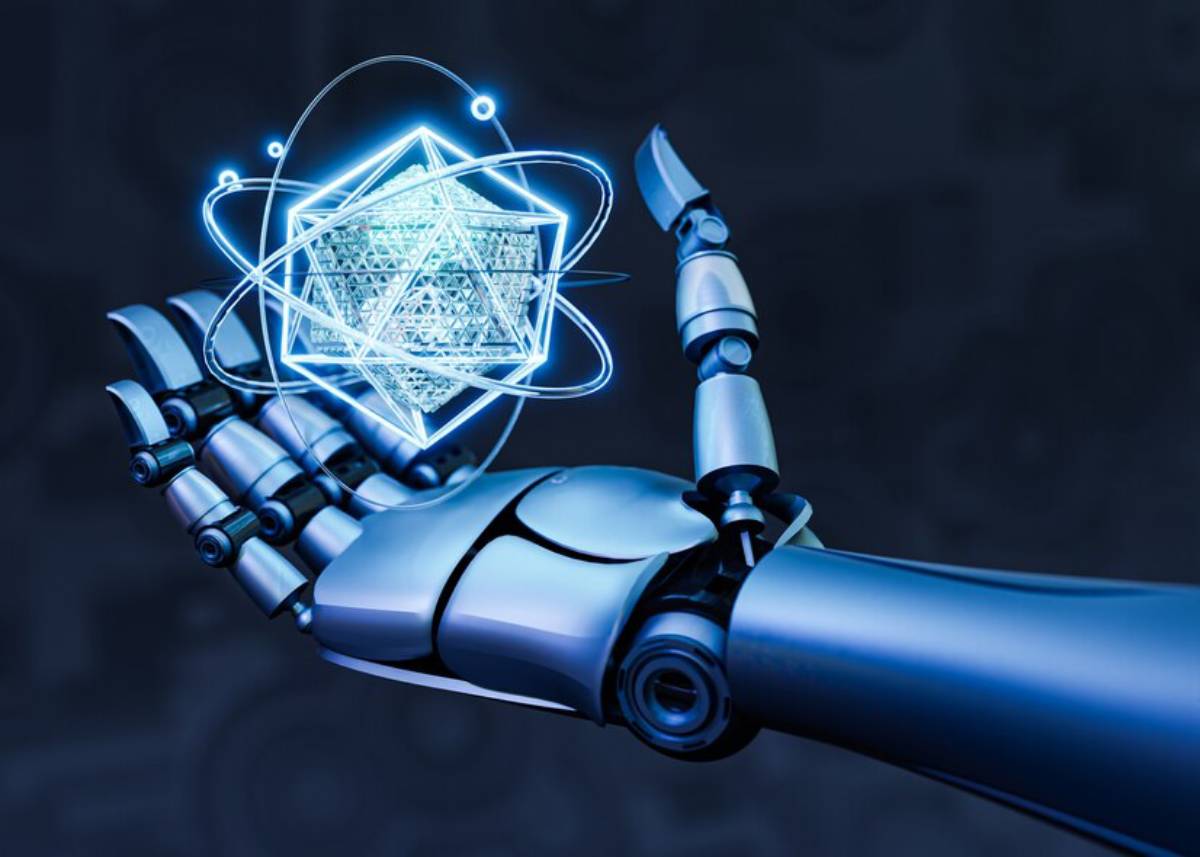
Neural Networks Powering the Next AI Breakthroughs
Artificial Intelligence is changing quickly. It is transforming how we live, work, and connect. Neural networks are the key framework behind many innovative and disruptive advances in AI technology. Inspired by the structure of the human brain, these complex systems are enabling machines to recognise patterns, interpret data, and learn from experience.
We are starting a new era in deep learning. Neural networks are getting smarter and more powerful. This progress brings breakthroughs in healthcare, self-driving cars, natural language processing, and more. In this article, we explore how neural networks work, where they’re headed, and why they are central to the next wave of AI innovation.
What Are Neural Networks?
Neural networks are algorithms that recognise patterns and relationships in data. They consist of layers of connected nodes, known as “neurons.” These neurons process input data and adjust their internal weights during training. This helps improve accuracy.
The Basic Structure:
- Input Layer: Receives the raw data.
- Hidden Layers: Perform transformations and computations.
- Output Layer: Produces the final prediction or result.
Each link between nodes has a weight. Nodes use activation functions to decide if they will send signals forward. Over time, the network “learns” by adjusting these weights to minimise errors.
This architecture allows neural networks to learn complex, non-linear relationships—making them ideal for tasks like image recognition, speech synthesis, and language translation.
The Power of Deep Learning
Deep learning is a subset of machine learning that uses neural networks with many hidden layers—hence the term “deep.” These deep neural networks (DNNs) can model intricate patterns in high-dimensional data, outperforming traditional algorithms in many applications.
Types of Deep Neural Networks:
- Convolutional Neural Networks (CNNs): Optimised for image and video analysis.
- Recurrent Neural Networks (RNNs): Handle sequential data like speech and text.
- Transformers: Revolutionising natural language processing and large language models.
- Generative Adversarial Networks (GANs): Create synthetic data, such as realistic images or audio.
Each of these architectures is powering some of today’s most significant AI developments.
Real-World Applications Driving Innovation
1. Healthcare and Medical Imaging
Neural networks are transforming the medical field with faster, more accurate diagnostics.
- Image analysis: Detecting tumours, fractures, or anomalies in MRIs, CT scans, and X-rays.
- Predictive analytics: Forecasting disease progression or hospital readmission risks.
- Drug discovery: Analysing molecular structures to identify potential treatments.
These tools enable earlier interventions, reduce diagnostic errors, and accelerate research—potentially saving lives.
2. Autonomous Vehicles
Self-driving cars use neural networks to understand sensor data and make quick decisions.
- Object detection: Recognising pedestrians, other vehicles, and road signs.
- Route planning: Navigating complex traffic environments.
- Sensor fusion: Integrating camera, radar, and LiDAR data.
Deep learning makes autonomous navigation safer and smarter. This is a big step for future transport systems.
3. Natural Language Processing (NLP)
Deep learning has greatly improved how humans and machines communicate. It’s changed everything from chatbots to language translation.
- Language models: GPT and BERT architectures can write essays, translate texts, and summarise articles.
- Speech-to-text systems: Powering tools like voice assistants and real-time transcription.
- Sentiment analysis: Helping brands understand consumer emotions and feedback.
These capabilities are central to everything from customer service automation to content creation.
4. Creative AI
Neural networks are also reshaping the creative industries.
- GANs generate artwork, music, or deepfake videos.
- AI tools assist designers, writers, and musicians with ideation and prototyping.
- Style transfer models blend artistic aesthetics with original content.
As the lines between human and machine creativity fade, AI is seen as a partner, not a rival.
5. Finance and Fraud Detection
Neural networks in banking and fintech analyse large datasets. They find anomalies and provide insights.
- Credit scoring and risk modelling
- Real-time fraud detection
- Algorithmic trading and portfolio optimisation
By identifying patterns in transactional data, AI systems improve financial decision-making and security.
Emerging Trends and Breakthroughs
As AI technology continues to evolve, so too do the neural networks that power it. Here are some of the cutting-edge developments defining the next generation of AI:
1. Self-Supervised and Few-Shot Learning
Traditional deep learning relies on vast amounts of labelled data. Self-supervised and few-shot learning try to lessen this need. They let models learn with little human help, just like we do.
This makes AI systems more efficient, adaptable, and scalable across industries.
2. Neuro-Symbolic AI
Neuro-symbolic systems blend neural networks’ pattern recognition with symbolic reasoning’s logic. This allows them to learn from data and make sense of it.
This offers:
- Better interpretability
- More robust problem-solving
- Safer AI decision-making
It’s a promising path toward more transparent and trustworthy AI.
3. Transformer Models and Foundation Models
Large deep learning models like GPT-4 and Google’s PaLM use huge data sets. They are then fine-tuned for different tasks.
These models can:
- Answer questions
- Write code
- Generate art
- Perform reasoning tasks
They mark a move towards general-purpose AI systems. These systems can manage many areas with little retraining.
4. Neural Architecture Search (NAS)
This is the process of automating the design of neural networks using AI itself.
NAS speeds up innovation and boosts performance by finding the best architectures quicker than humans. This cuts down on the need for expert help in designing models.
5. AI at the Edge
Neural networks are now being used on edge devices like phones, sensors, and wearables, not just in the cloud. This shift allows:
- Faster processing
- Enhanced privacy
- Reduced bandwidth use
This shift supports applications in healthcare, agriculture, and IoT-enabled environments.
Challenges and Considerations
Despite their potential, neural networks are not without limitations.
1. Black Box Problem
It’s often difficult to understand how a neural network makes decisions. This lack of transparency can cause problems in important fields like healthcare and law.
2. Bias and Fairness
If training data contains bias, the model will perpetuate it. Addressing bias is essential for creating equitable AI systems.
3. Resource Consumption
Training deep models needs a lot of computing power. This raises worries about energy use and its effect on the environment.
4. Security and Adversarial Attacks
Neural networks can be easily tricked by specific inputs. These inputs may lead to unexpected behaviour. Ensuring robustness is critical for safety.
The Future of AI Technology: What’s Next?
As neural networks grow stronger and more efficient, we get closer to achieving Artificial General Intelligence (AGI). This type of AI can understand, learn, and use knowledge across many tasks.
Future applications may include:
- Personalised AI tutors and coaches
- Real-time language and cultural translation
- AI collaborators in scientific research
- Robotics with human-like perception and adaptability
All these breakthroughs rely on ongoing improvements in deep learning and its neural networks.
The Path Forward
Neural networks power today’s top AI features. They enable everything from data analysis to human conversation, creativity, and mobility. As AI becomes a bigger part of our lives, it’s important to understand and improve these systems.
We’re entering a world where AI doesn’t just automate tasks—it augments human potential. Deep learning drives this change, thanks to smarter neural networks.
Act now: Check out a neural network tool, discover how models are trained, or keep up with the latest AI research. The future’s breakthroughs are happening now, driven by networks that think, learn, and grow.