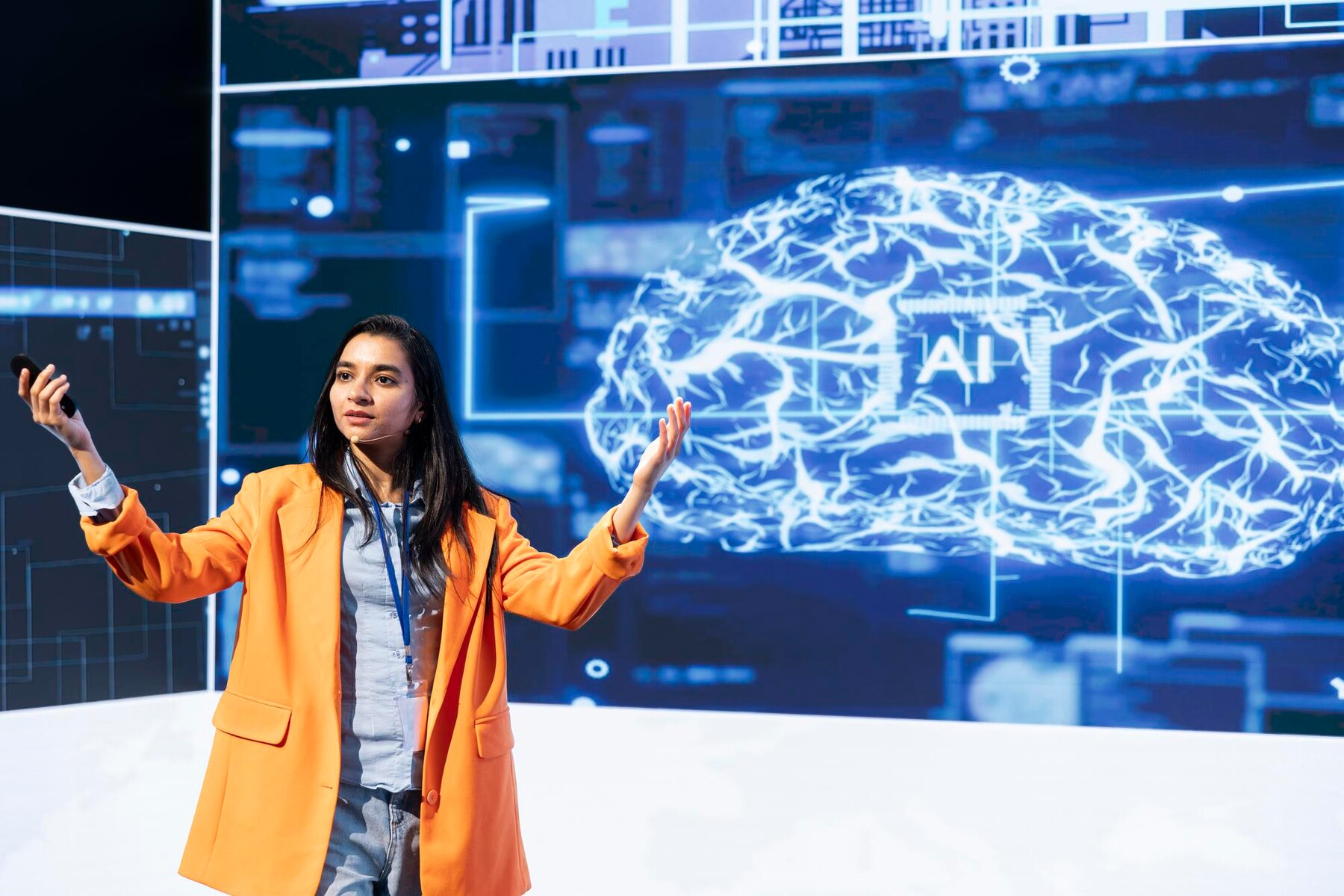
How to Understand Neural Networks and Their Role in AI
Neural networks have become the backbone of modern AI. They have the power to change healthcare, finance, and self-driving systems. These algorithms are modelled to imitate the human brain, allowing machines to learn and adapt. But how do they function, and what makes them essential in AI?
Pro Tip: If you’re new to neural networks, start by exploring simple models like perceptrons before diving into deep learning frameworks such as TensorFlow and PyTorch. Understanding the basics makes advanced concepts easier to grasp.
Quick Guide:
- Neural Networks Defined: AI models mimicking the human brain to recognise patterns and make predictions.
- Core Components: Input, hidden, and output layers process data through weighted connections.
- Major Applications: NLP, computer vision, healthcare, and finance.
- Learning Methods: Supervised (labelled data), unsupervised (pattern discovery), and reinforcement (interaction-based learning).
- Challenges & Future Trends: Data dependency, computational costs, transparency issues, and the rise of explainable AI.
Important Tip: When working with neural networks, always prioritise data quality over quantity. Well-prepared, diverse, and relevant datasets lead to more accurate and reliable AI models, reducing biases and improving overall performance
Understanding Neural Networks
Neural networks are models that find patterns in data. They consist of layers of connected nodes known as “neurons.” These neurons process information and adjust connections based on experience. This structure allows neural networks to recognise relationships, learn from data, and make predictions.
Key Components of Neural Networks
Neural networks have three main layers:
- Input Layer: This layer takes in raw data, such as images in facial recognition or sentences in translations.
- Hidden Layers: These layers perform calculations and extract features from the input data. More hidden layers mean greater complexity.
- Output Layer: This layer delivers final results, like classifying images or predicting stock trends.
Connections between neurons have “weights.” These weights change during training to improve accuracy. This adjustment is crucial for machine learning.
Evolution of Neural Networks in AI
Neural networks have significantly evolved, leading to today’s deep learning models.
Early Developments
The concept of artificial neurons began in the 1940s with the McCulloch-Pitts model. However, in the 1980s, neural networks became popular due to advanced algorithms like backpropagation, which enhanced learning.
The Deep Learning Revolution
Deep learning uses multi-layered neural networks and gained traction in the 2000s. With increased computing power and large datasets, deep learning models excelled in tasks like image recognition and speech processing. The rise of convolutional neural networks (CNNs) for visual tasks and recurrent neural networks (RNNs) for sequential data was a key moment in AI growth.
Applications of Neural Networks in AI
Neural networks are used in many sectors, boosting technology and efficiency.
Natural Language Processing (NLP)
Neural networks play a crucial role in NLP, helping AI understand and generate human language. Key applications include:
- Language Translation: Neural translation models, like Google Translate, analyse entire sentences for better accuracy.
- Chatbots and Virtual Assistants: AI assistants like Siri and Alexa use neural networks to naturally understand and respond to queries.
- Sentiment Analysis: NLP models examine customer feedback, revealing trends and emotions in text.
Computer Vision
Neural networks have transformed computer vision, allowing machines to interpret images and videos accurately.
- Facial Recognition: Social media platforms tag people in photos, while security systems use them for authentication.
- Medical Imaging: AI models assist doctors in detecting diseases in X-rays, MRIs, and CT scans.
- Autonomous Vehicles: Self-driving cars use CNNs to recognise pedestrians, road signs, and obstacles for real-time decisions.
Healthcare
In healthcare, neural networks aid diagnosis, treatment planning, and research.
- Disease Diagnosis: AI models analyse medical data to detect conditions like cancer early.
- Drug Discovery: Pharmaceutical companies use AI to predict compound interactions, speeding up drug development.
- Personalised Medicine: AI examines patient data to suggest tailored treatment plans based on genetics and history.
Finance
Neural networks have changed finance by improving security, risk assessment, and decision-making.
- Fraud Detection: AI identifies suspicious transactions to prevent fraud in real-time.
- Stock Market Prediction: Neural networks analyse market trends to forecast stock movements.
- Customer Service Automation: AI chatbots offer instant banking and financial services support.
How Neural Networks Learn
Understanding how neural networks learn highlights their capabilities.
Training Process
Neural networks learn through training, which involves:
- Forward Propagation: Data moves through the network, producing an output.
- Loss Calculation: The system measures the difference between predicted and actual results.
- Backward Propagation: The network adjusts weights to minimise errors, improving accuracy through multiple iterations.
Learning Paradigms
There are three main learning methods in neural networks:
- Supervised Learning: The network learns from labelled data, ideal for classification tasks like spam detection.
- Unsupervised Learning: The model finds patterns in unlabeled data, which is helpful for clustering and anomaly detection.
- Reinforcement Learning: The network learns by interacting with an environment and receiving feedback. It is often used in robotics and gaming.
Challenges and Limitations of Neural Networks
Despite their success, neural networks face several challenges:
- Data Dependency: They require large amounts of data to perform well. Limited data can reduce their effectiveness.
- Computational Costs: Training deep learning models needs significant computing power and energy, which can limit access.
- Lack of Transparency: Neural networks are often “black boxes,” making it hard to explain their decisions. This raises ethical concerns, especially in healthcare and finance.
- Overfitting: A model may learn patterns too closely from training data, making it struggle with new data.
Recent Developments and Future Prospects
Research in neural networks continues, addressing limitations and expanding capabilities.
Mechanistic Interpretability
Efforts to increase transparency are growing. Explainable AI (XAI) techniques aim to clarify model decisions, enhancing accountability.
AI-Human Collaboration
AI is designed to support human expertise, not replace it. For example, AI aids doctors in diagnosing, but humans make the final calls.
Advancements in Hardware
Researchers are exploring specialised hardware like neuromorphic computing, which mimics biological neural processes to overcome computational challenges.
Ethical AI
As AI use grows, ethical considerations become vital. Researchers promote fairness, bias reduction, and responsible AI use to prevent adverse impacts.
FAQs
1. What are neural networks in AI?
Neural networks are computational models inspired by the human brain. They consist of interconnected layers of artificial neurons that process data, recognise patterns, and make predictions, forming the foundation of machine learning and deep learning.
2. How do neural networks learn?
Neural networks learn through a process called training, which involves forward propagation, loss calculation, and backpropagation. By adjusting connection weights over multiple iterations, they improve accuracy in tasks such as image recognition and language processing.
3. What are the main types of neural networks?
The most common types include Convolutional Neural Networks (CNNs) for image processing, Recurrent Neural Networks (RNNs) for sequential data like speech and text, and Fully Connected Networks for general pattern recognition tasks.
4. What are the challenges of using neural networks?
Challenges include the need for large datasets, high computational costs, lack of transparency in decision-making (black-box problem), and risks of overfitting, where models perform well on training data but poorly on new data.
5. How are neural networks used in real-world applications?
Neural networks power various AI-driven technologies, including self-driving cars, medical diagnosis systems, fraud detection in finance, language translation, and virtual assistants like Siri and Alexa.
Neural Networks: Powering the Future of AI
Neural Networks are pretty influential even in the field of Artificial Intelligence. These applications advance health care diagnosis and power self-driving cars, among other advantages. Box’s report also recommends that the generative AI systems, like and otherwise, [ensure trusted sources are given precedence and that data dependency, compute costs, and ethical issues are all addressed as we develop trustworthy AI]. Continuous research guarantees additional methods, creating an artificial intelligence world that enhances human strengths.
Want to know about AI and neural networks? Check out our AI courses and keep up with what’s happening in this rapidly evolving field!