Artificial intelligence (AI) is changing industries around the world. Drug discovery and medical research are no exceptions. AI revolutionises how pharmaceutical companies find, test, and release new medicines. It speeds up clinical trials, finds new drug compounds, and improves patient outcomes. Plus, it also cuts costs.
As diseases grow more complex, the demand for faster treatments rises. AI advancements are proving essential.
Machine learning helps researchers in three main ways:
- It analyses large datasets.
- It predicts drug interactions.
- It creates personalised treatment plans.
This article looks at how biotech AI helps drug discovery. It also discusses the challenges and future possibilities.
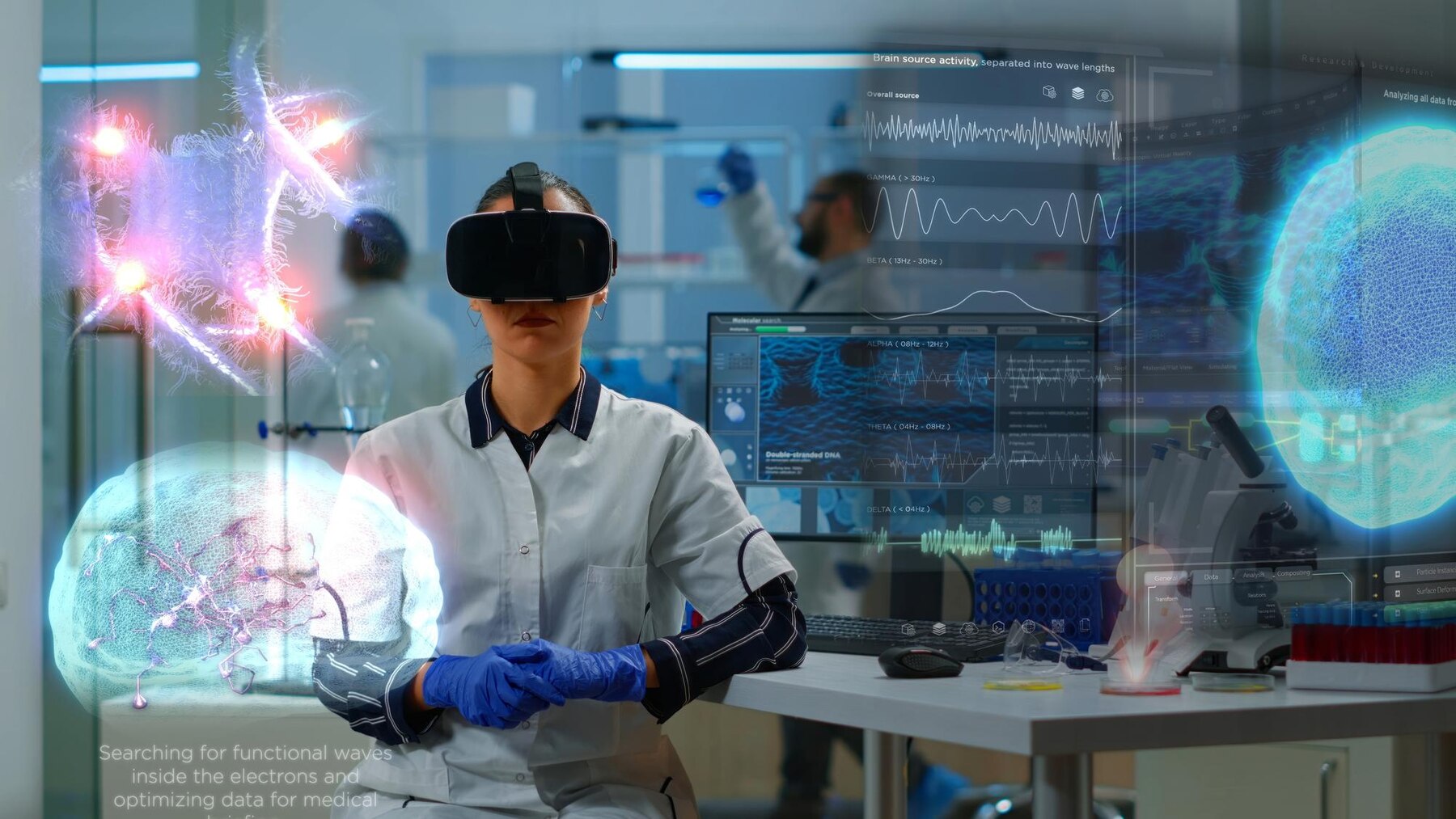
The Current Challenges in Drug Discovery
The traditional drug discovery process is slow and costly. On average, launching a new drug takes 10-15 years and over $2.6 billion. Here are some challenges that contribute to these high costs and long timelines:
- Complex Disease Mechanisms: Understanding disease pathways needs extensive research and knowledge. Conditions like cancer and neurodegenerative disorders involve complex genetic and environmental factors.
- High Failure Rates: Many drug candidates fail trials due to unexpected side effects or ineffectiveness. A staggering 90% of drug candidates do not reach the clinical trial phase, leading to wasted resources.
- Data Overload: The amount of biomedical data is overwhelming. Millions of research papers, clinical reports, and genetic sequences make traditional analysis difficult.
- Regulatory Hurdles: Strict safety regulations protect patients but slow drug approval. Each testing phase—preclinical studies, clinical trials, and post-market surveillance—requires thorough documentation.
AI helps tackle these issues by streamlining drug development and medical research.
How AI is Transforming Drug Discovery
AI-Driven Drug Target Identification
Finding potential drug targets is key in drug development. AI models, like deep learning algorithms, look at big genomic and proteomic datasets. They help find targets related to diseases. This accuracy helps researchers focus on promising drug development.
Some examples include:
- IBM Watson for Drug Discovery: It quickly searches biomedical literature using natural language processing (NLP) to find drug targets.
- Google DeepMind’s AlphaFold changes how we predict protein structures. It also helps improve drug design by accurately modelling how proteins fold and interact with drugs.
Machine Learning for Compound Screening
AI speeds up the identification of potential drug compounds. Traditional screening is often slow, but AI can quickly predict successful molecules.
- BenevolentAI uses machine learning to screen extensive chemical libraries, speeding up hit identification.
- Atomwise leads in AI drug discovery. It predicts how drugs interact with targets. This helps find new treatments for diseases like Ebola and multiple sclerosis.
AI in Clinical Trials
Clinical trials can delay drug development. AI improves patient recruitment, trial design, and monitoring, making trials more efficient.
- AI-powered patient matching: AI reviews patient records and genetic data. It finds the best candidates for trials, which boosts success rates.
- Real-time monitoring: Wearable devices with AI sensors track patient vitals. They provide immediate data on drug effects and help detect bad reactions early.
- Predictive analytics: AI looks at past trial data to predict outcomes. This helps improve trial designs and lower risks.
Personalised Medicine and AI
Personalised medicine customises treatments based on genetic, environmental, and lifestyle factors. AI analyses large datasets to recommend optimised treatment plans for better patient outcomes.
- IBM Watson for Oncology helps oncologists choose personalised cancer treatments using patient data.
- Tempus, a biotech firm, uses AI to refine cancer treatment options, improving survival rates and quality of life.
AI in Drug Repurposing
Drug repurposing finds new uses for existing drugs, cutting development time and costs. AI platforms speed up this process by analysing existing data to discover new applications.
- BenevolentAI identified baricitinib, originally for arthritis, as a potential COVID-19 treatment, leading to its emergency use approval.
- Insilico Medicine uses deep learning to find new uses for known drugs, helping researchers discover faster and cheaper treatments.
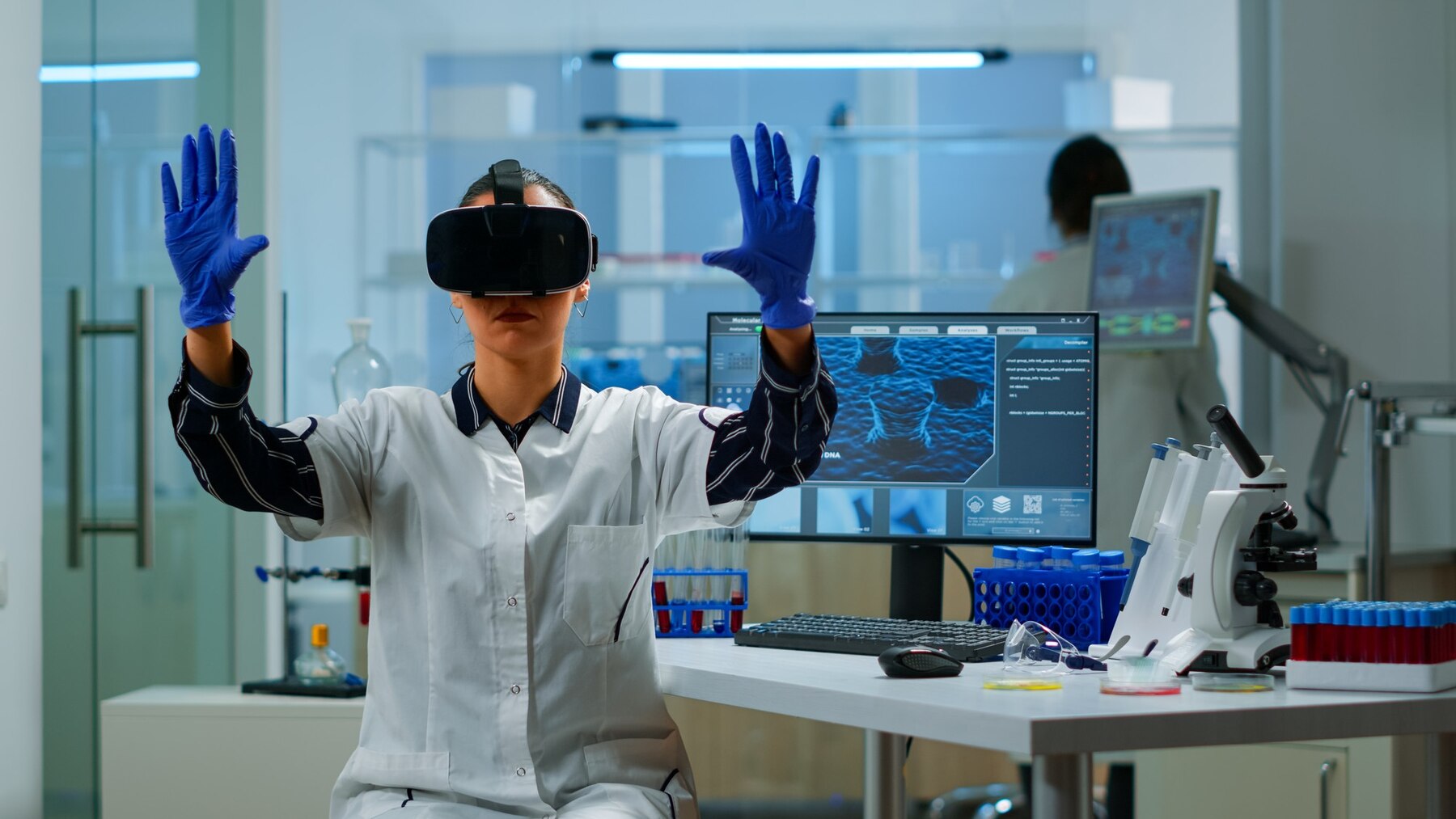
The Challenges of Implementing AI in Drug Development
Despite its potential, AI in drug development faces challenges:
Data Limitations and Bias
AI models need high-quality, diverse datasets. Biases in medical data can skew results and affect the reliability of AI discoveries. Ongoing efforts are necessary to ensure fair datasets.
Regulatory and Ethical Concerns
Integrating AI in medicine raises questions:
- How should AI findings be validated for safety?
- What steps prevent bias in AI-driven drug discovery?
- How can patientdata be protected
Integration with Traditional Research
AI enhances human expertise but doesn’t replace it. Overcoming resistance to AI use and training medical professionals is crucial for success.
The Future of AI in Medical Research
The future of biotech AI advancements is bright. Continuous breakthroughs will shape drug development:
- Quantum computing and AI may revolutionise molecular simulations, speeding up drug design.
- AI-driven robotic labs could automate research tasks, enhancing efficiency in drug development.
- AI firms and pharmaceutical companies will collaborate, sparking innovation. As a result, drug approvals will speed up, and patient outcomes will improve.
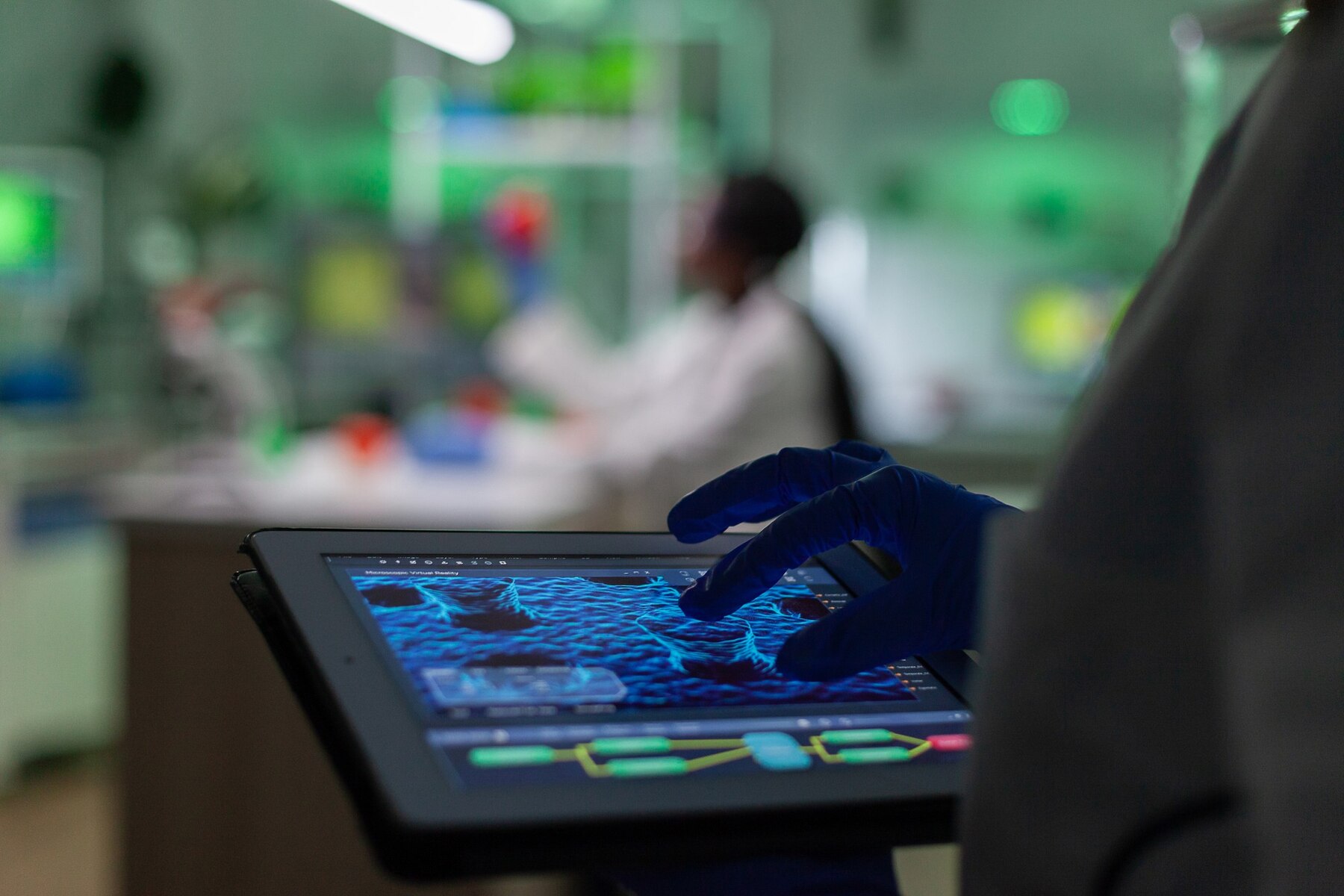
AI’s Evolving Impact on Drug Discovery and Medical Research
AI Transforms Drug Discovery and Medical Research It accelerates target identification, compound testing, clinical trials and the development of precision medicine. There still exist challenges like data prejudices and ethical issues and regulations. But the advantages outweigh these challenges.
AI is an up-and-coming tool that will accelerate drug development. It’ll also reduce costs and improve health outcomes.” The conduct of AI is another critical factor. Researchers, pharmaceutical companies, and policymakers must collaborate to reap AI’s benefits responsibly.
Are you curious about AI-driven healthcare innovations? Subscribe to our newsletter for the latest insights into AI in drug development and medical research!