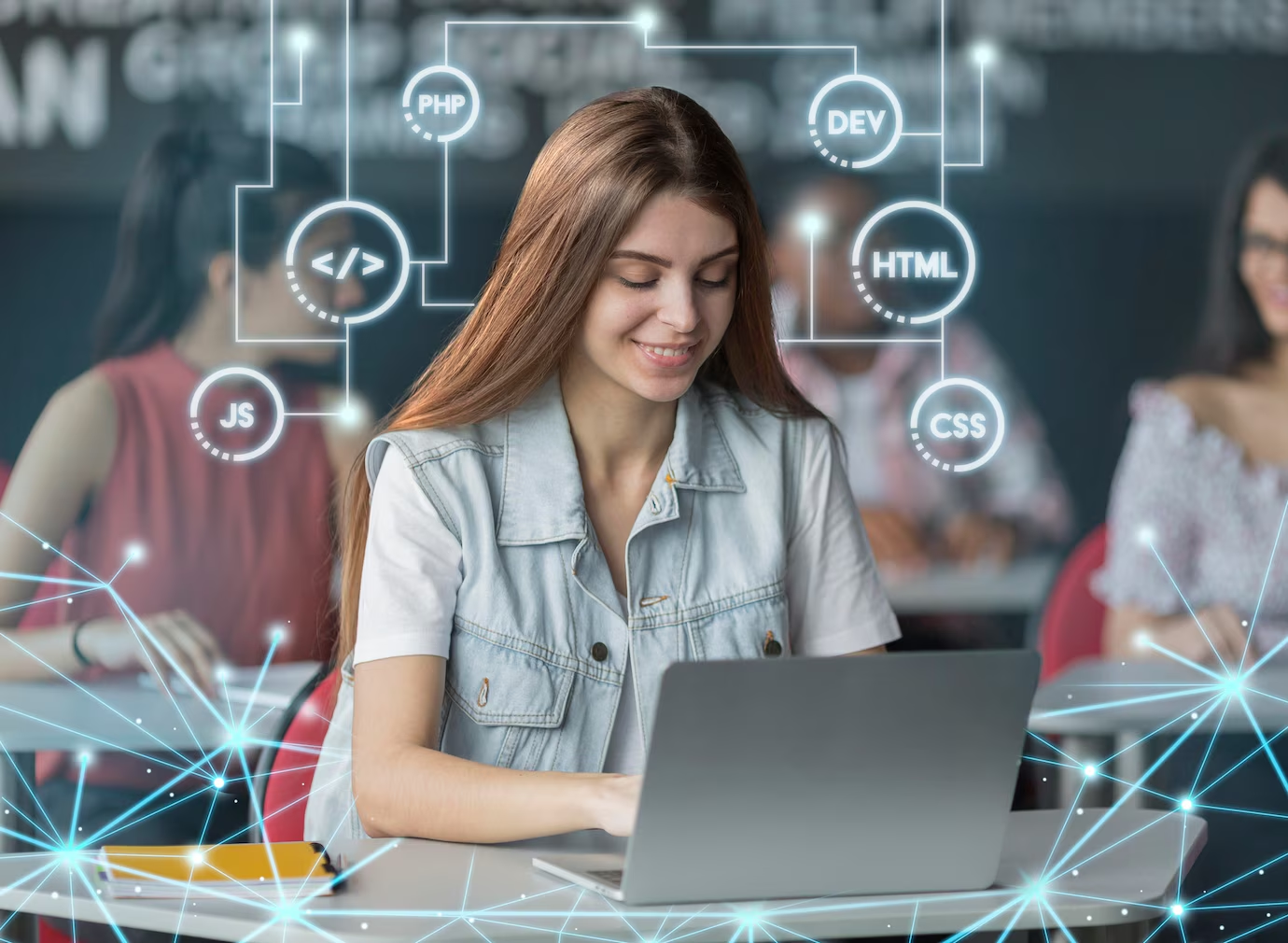
How Reinforcement Learning is Driving AI Innovation
Artificial Intelligence (AI) is growing quickly. Reinforcement learning (RL) is changing how machines learn and make decisions. Reinforcement learning is different from traditional supervised learning. In supervised learning, AI needs labelled data. However, with reinforcement learning, AI learns through trial and error, just like humans do. This adaptability changes industries like robotics, finance, gaming, and more.
In this article, we will cover:
- What reinforcement learning is and how it differs from other AI methods
- Key reinforcement learning applications across industries
- The role of robotic decision-making AI
- The future potential of reinforcement learning
What is Reinforcement Learning?
Reinforcement learning is a type of machine learning where an agent learns by interacting with an environment. The aim is to take actions that increase rewards over time. The RL model has three main parts:
- Agent: The decision-maker
- Environment: The setting where the agent operates
- Rewards: Feedback that helps guide learning
How Reinforcement Learning Differs from Other AI Methods
RL is different from supervised learning. In supervised learning, AI uses pre-labelled datasets. In contrast, RL focuses on learning from experience. Key differences include:
- No labelled data: RL agents learn via trial and error, not fixed labels.
- Decision-making focus: While supervised learning predicts outcomes, RL aims for long-term strategies.
- Dynamic adaptation: RL can better adapt to changing environments than traditional models.
Key Reinforcement Learning Applications
Reinforcement learning is making a significant impact in many industries. Here are some critical applications.
Robotics and Autonomous Systems
A significant advancement in AI is using RL in robotics. RL allows robots to:
- Improve decision-making, helping them navigate and interact with the real world.
- Learn complex tasks like grasping objects, assembling parts, and walking alone.
- Adapt to unexpected challenges without human help. Case Study: Boston Dynamics’ Spot Robot
Boston Dynamics uses reinforcement learning in Spot, a four-legged robot. Spot moves through changing terrains. With RL, Spot can avoid obstacles, keep balance, and perform tasks effectively.
Finance and Trading
Reinforcement learning is transforming algorithmic trading. Here, AI makes trades using market data. Key applications include:
- Portfolio optimisation: AI can adjust assets based on risk and reward.
- Fraud detection: RL models find anomalies and fraud.
- Market simulation: AI mimics trading situations to enhance investment strategies. Deep Q-Networks, for example, are used in stock trading.
Financial firms use Deep Q-Networks (DQN) to discover the best trading strategies, which reduce risk and increase returns.
Healthcare and Drug Discovery
In healthcare, RL is enhancing personalised medicine and drug discovery by:
- Improving treatment recommendations based on patient feedback.
- Optimising robotic surgeries for precision.
- Speeding up drug discovery by testing compounds through AI simulations.Notable Development: AlphaFold
DeepMind’s AlphaFold uses AI and reinforcement learning to predict protein structures. This breakthrough is changing medical research.
Gaming and Entertainment
The gaming industry has been a testing ground for reinforcement learning. AI bots now beat human players in complex games like:
- Chess and Go: DeepMind’s AlphaGo won against world-champion players using RL.
- Video games: AI learns to play games like Dota 2 and StarCraft II through self-play reinforcement learning.
- Character development: AI-powered NPCs now act more like humans in open-world games.
Smart Transportation and Traffic Management
In smart cities, RL improves traffic control and autonomous vehicle navigation.
- Self-driving cars: Companies like Tesla and Waymo use RL for vehicle autonomy.
- Traffic flow optimisation: AI signals adapt to reduce congestion.
- Predictive maintenance: AI looks at sensor data to stop transport breakdowns. Case Study: Waymo’s Self-Driving AI.
Waymo uses deep reinforcement learning to enhance vehicle decision-making, boosting safety and efficiency.
The Role of Reinforcement Learning in Robotic Decision-Making AI
Reinforcement learning is crucial for robotic decision-making. It allows machines to operate on their own in complex environments. Important aspects include:
- Autonomous control: Robots learn to adapt without needing human help.
- Sim-to-real transfer: Training in simulations before real-world deployment.
- Continuous learning: Robots improve their skills over time through feedback.
Industrial Automation
Many factories are using AI-driven robots for assembly lines. Tesla uses RL-powered robots for precise manufacturing, which reduces errors and boosts efficiency.
AI-Powered Assistive Robots
Reinforcement learning is also used in assistive robots, which support the elderly and disabled. These robots learn to help with daily tasks like:
- Medication reminders
- Mobility assistance
- Conversational companionship
The Future of Reinforcement Learning
Reinforcement learning is constantly changing, with exciting prospects:
- AI-human collaboration: RL-based AI will collaborate with humans in medicine and creativity.
- Multi-agent RL: Systems with many AI agents will achieve new levels of intelligence.
- Energy efficiency: AI can improve renewable energy use to cut carbon footprints.
Ethical Considerations
As reinforcement learning progresses, ethical issues arise, such as:
- Bias in AI decision-making
- Job loss due to automation
- Ensuring AI safety in critical areas
The Impact of Reinforcement Learning on AI Advancement
Reinforcement learning is changing AI. It’s driving significant improvements in robotics, healthcare, finance, and other areas. As AI evolves, RL will be central to driving innovation across industries.
Reinforcement learning is changing many fields. It improves trading strategies, drives self-driving cars, and transforms medical research.
Want to learn more about reinforcement learning and AI innovation? Stay updated on the latest AI trends by subscribing to our newsletter or joining the conversation in our tech forums!
Businesses and researchers can use these insights to unlock the full power of reinforcement learning. This could lead to the next wave of AI breakthroughs.